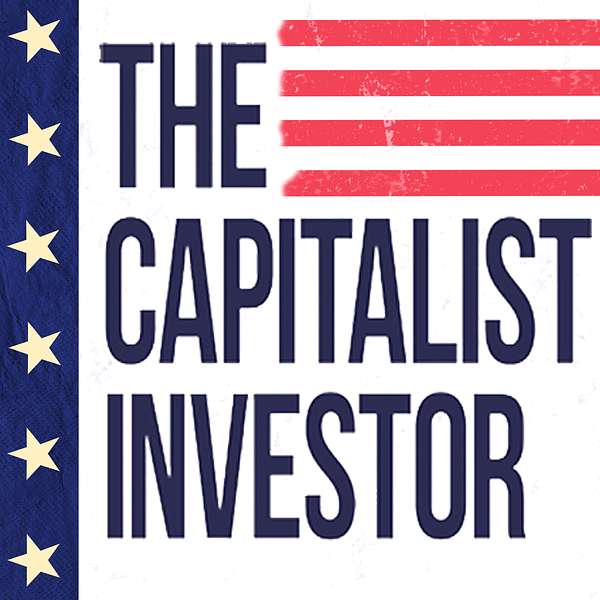
Capitalist Investor
Check out the "Capitalist Investor" podcast where hosts Derek, Luke and Tony break down complex financial topics and recent market trends with a sharp eye. This podcast is all about getting into the nitty-gritty of things like stock buybacks, tax policies, meme stocks, and a whole lot more. The guys aren’t just brains; they keep things light with a great mix of deep dives and easy banter that keeps you hooked and learning. Whether they’re chatting about Warren Buffett’s latest strategies, how Biden’s tax plans might hit different income levels, or the buzz around a big golf tournament, you’ll come away with a solid grip on how these issues could shake up your financial world. Perfect for investors, retirees, or just anyone keen to keep up with the financial universe, "Capitalist Investor" makes the complex understandable and entertaining.
Capitalist Investor
Navigating AI Patents: Key Insights and Challenges, Ep. 253
The latest episode of the "Capitalist Investor" podcast featured hosts Tony and Luke with special guest Mike Hudzinski, a prominent patent attorney from Tucker Ellis LLP in Cleveland. The trio delved into the intricate world of AI, patents, and the broader economic landscape. Here are five hot topics from the episode that shed light on the future of technology, law, and the economy.
1. Patentability of AI Algorithms: The Human Element
One of the primary discussions revolved around the complexities of patenting AI algorithms. Mike Hudzinski clarified that “you can't patent a naked algorithm because it can be performed by a human.” For an algorithm to be patentable, it must produce a result that could not be achieved manually with pen, paper, and a calculator. This distinction underscores the need for a significant innovation component to secure patent rights.
2. Obviousness in Software Patenting: Novelty vs. Patentability
The conversation moved to the difference between novelty and patentability in software. Tony and Mike discussed how small changes in code, such as a slight alteration within a complex algorithm, might not meet the threshold for patentable innovation. The key lies in whether these changes are obvious to an ordinary programmer. If a minor adjustment leads to a significant improvement, it might be considered for patenting, otherwise not.
3. AI Writing Its Own Code: Legal Implications
As AI technology advances, there's growing curiosity about whether AI can patent its self-generated code. Mike pointed out new federal regulations stipulating that patents must be created by humans. According to United States code, the claimed invention needs to be human-made, thus keeping AI-generated inventions outside patent eligibility. This maintains a critical human oversight in the innovation process.
4. Errors and Reliability of AI: Real-World Consequences
A discussion on the reliability of AI unraveled some of its limitations. Tony referred to an incident where an attorney cited erroneous legal information generated by AI, leading to significant problems. Mike emphasized that both professionals and students must verify AI-generated outputs. Generative AI, although powerful, relies on the data it has been trained on and is prone to inaccuracies, which can have far-reaching consequences in critical fields like law.
5. Economic Impact of AI on Jobs: A Double-Edged Sword
The episode concluded with a preview of upcoming discussions on the economic impact of AI, particularly job displacement. While AI is poised to enhance efficiency and productivity in various sectors, it could also lead to job loss. As Tony noted, the implementation of AI might result in what seems like "job destruction." The conversation will continue in future episodes, exploring the balance between technological advancements and the preservation of the job market.
The Capitalist Investor podcast continues to provide valuable insights into how emerging technologies intersect with legal frameworks and economic realities. Stay tuned for upcoming discussions, including public vs. private sector job dynamics and the impact of electric vehicles (EVs) on the economy.
For those seeking to navigate these complex issues, the episode reaffirms the importance of staying informed and consulting with qualified professionals. Whether you're an investor, a tech enthusiast, or just curious about the future, these conversations are crucial for understanding the rapidly evolving landscape.
**Disclaimer:** The opinions expressed in the podcast are for general informational purposes only and are not intended to provide specific advice or recommendations for any investment, legal, financial, or tax strategy. Please consult a qualified professional about your individual needs.
Welcome to this week's episode of the Capitalist Investor. As always, you have diamond Hands D, Tony the Tiger. You got me, cool Hand Luke. And we actually have a special guest today, Mike Kudzynski. He is one of our clients, also a patent attorney by trade. Mike, give us a quick intro. Thanks for being here. Thanks for having me. Gentlemen. My name is Mike Kudzinski. I'm with the law firm Tucker Ellis LLP in Cleveland. I'm a patent attorney there specializing in software patents, electrical and electromechanical patent work. Yeah. Well, we're excited to have you for the next week on our next three episodes, this one and next two. A lot of things in the AI tech space. They all need patents, they all need someone attorney looking over what's going on, especially in this innovative world we're living in, this environment we're heading into. So today we're going to focus on that kind of AI aspect that we always hear around the news cycle. I feel like we can't run away. Everyone's talking about Nvidia AI chips, the future of what that looks like. And one thing I was talking to you about, Mike, before, is I was trying to patent algorithms back in the day for trading the financial markets. And I liked one of the things you were telling me before about how there's certain things you can patent or can't patent around an algorithm. Can you tell us a little bit about that? Because maybe there's certain listeners out there that are listening to this that want to get involved in the AI space, and they're like, maybe I have this cute, crazy good thing, and I just don't know if I can patent it. That's right. You can't patent a naked algorithm, basically because it can be performed by a human. So essentially you would be patenting a human who would be performing that algorithm. So the algorithm needs to have some sort of extra result that would happen by virtue of executing the algorithm. That couldn't happen basically by a human using paper, pen, pencil and calculator. Yeah, I mean, I remember when I was going through engineering school, I went through C programming. I don't even know if that's probably obsolete at these days. But you were writing code. These codes can get extremely complicated and very long. That's right. And all you need to do is change one minus sign or a plus sign, and it's different. It's different, right. So that's different. So another reason why you really can't patent, it's like. And plus, you always want to increase it, not increase it, but make it better. And so, like, is version two then patent? And, like, you just have to keep on making patent. Like, help me understand that. Okay, so you're talking about the difference, really, between novelty and patentability. So there's two different pieces of software, could be novel relative to each other, but does the difference rise to the level of a patentable difference? Is that an invention to make a small, minor change to the software from one version to another to rise to warrant federal patent right protection? And that question is answered basically based on obviousness. Would version two be obvious in version one to an ordinary programmer of ordinary skill in the gap? So attorney. Put that at 10,000 foot. Level so we can talk with attorneys, and they're like, whoa, when you said. Two lines of code, I'm guessing it's not patentably distinct. It could be novel because it's two pieces of code. Now, if you tell me that those two pieces of code result in a 20% difference in time required to process the data, you know, okay, now you have some kind of result that might not be obvious. Well, the result speaks to whether the change would have been obvious to one of ordinary skill in the art, and the ordinary skilled person would be another programmer, maybe in another company. And the question is then why? Why wouldn't that person in that company have made the same change? Because it's not obvious. Right. But you could. You could point to the net result of that small change in the output. What if. Okay, here's one thing I'm really wondering about. We haven't got to the point where AI can program itself. All right, what if we get to the point where AI starts to program itself, write its own code? Can technology itself be patentable when technology writes it? Yeah. So there's some new federal regulations that I've been looking at, and the answer is going to be no. There has to be a human involved. And the United States code, 35 USC, says it has to be basically a human. Human that writes it. Yeah, a human that writes it and implements it. The claimed subject matter has to be invented by a human. I mean, if I spent a week, a month, a year writing some code that changed the world, I'd want to patent it. Yeah. I mean, like, and. But like I said, all somebody would need to do is take that thousand page of code and change a plus two or minus. It does something different, and now it's obsolete or changed or. That's right. Not patented again. That's right. And technology moves really upset because 99.999% of that is all of me. And I got. Got blown out of the water. That's what I was. Well, but, but what I'm also saying is that, is that incremental difference, the little incremental difference itself separately patentable. And I would suggest it might not be for the. Your competitor who made that change, is what I think the. Right. Yeah. And it's kind of like, you know, you know, generic soap or something. Right. That's right. I'm gonna steal, you know, Perel's whatever thing and change an ingredient, and now it's mine. Yeah. Sort of like reverse engineering somebody else's work. Yeah. Trying to improve upon it. Improve upon it. Or just change it slightly. So it's not the. Quote, unquote the same. Right. That's right. To change it slightly is to try to avoid infringement of the patent owners rights. So we've talked about, like, we go down these conspiracy theory paths. Here we go. It's so fun. And here we go. So let's talk about the errors of AI. Cause, I mean, we can talk about how it's gonna implement the economy, and I can spin that, too. But the errors. So one of the talks that we had a few podcasts ago, there was an attorney that got in trouble. I don't know if he was like. Again, I don't remember, like, if it was, like, an attorney going through law school or an attorney that was, like, writing a case, and they dug up something through AI, and AI changed. Like, they pulled up the case and changed the verdict to, like, make it. He was quoting a case, and it was all wrong. Like, he changed the AI, changed the verdict, and the guy ran with it, and something like, dude, that was not how that case ran out. Like, where'd you get this information? That's right. I got it from this computer. So, I mean, it's still not perfect, right? It is not perfect. And students do the same thing with term papers. And so, you know, they're found out some images that are produced, you know, using generative AI. Right. They are incomplete. You have, you know, three fingered models and people with, you know, partially formed whatever, because they're collecting data from a model and putting together some sort of thing that's generated from maybe an incomplete or inaccurate data set. Right. And so that's kind of what happens. And when attorneys rely upon it, it's kind of bad. What you're supposed to do is go back and they called spade the brief, which is, you know, look up that case and see if I check it. Yeah, I think students have the same problem, like I said. Yeah, well, it goes back to the copyright kind of thing. You know, you said technology can't patent technology, that if technology writes, it has to be human. The same thing with copyright. I've heard that kind of philosophy is the same, and that kind of is becoming kind of the norm too, where you have to have a human write something to be copyrightable. Yeah, that's right. Okay. That's right. It's interesting because, you know, people are using chat GBD to write term papers or to write books even. I mean, you can write a hundred page book now by typing in just a couple prompts. And if that's the case, everyone's gonna be writing their own book, right. And, you know, we were gonna kind of, we lead into like, how AI implements into the economy, you know, legal patents, technology, manufacturing. Right. And I can understand that. It makes things faster, more efficient, cost efficient. To me, honestly, that sounds like job destruction. It honestly, it's going to eliminate jobs is. Well, I think that's a conversation for our next podcast. Come up here in a couple days when we're talking about actually public sector versus private sector jobs, right? That's right. That's right. But I do think that's touched on it quickly on this, and we go deep, dig deeper on the next one. I think that is absolutely gonna happen. And plus, you know, especially if one company owns a certain patent, I mean, can't you like, be almost a monopoly on top of that? And then that also goes into the job market. Yeah, well, patents are a monopoly for a finite term. And so you have the right to exclude others from making, using, selling, importing the thing that is patented. So you do have a personal or, you know, your company has an entity right in that monopoly. And the way the patent system works just overall, is that for, in exchange for disclosing your invention to the public, which it is, it's published, you have the right to exclude others from using, making, using, selling, or importing the thing that's patented. So it is a monopoly. What's it, what's the shelf life on a patent? Like 30, 20 years from filing, 20 years. So, like Nvidia, like, some of their patents, I'm sure they have thousands. They have thousands of patents. Yeah. Like, basically they could, if they have a breakthrough technology, they could be the main player in the game for 20 years on that one. Technology. That's right. But technology moves forward. Right. And I was like, you change a. Little bit of a code and it's different. Right. That's right. And I actually, I'm not sure who Nvidia's competitors are. And it's interesting to look at what kind of foundries do we have in this country that could compete with Nvidia moving forward with new sort of processor capabilities? Why they're so big now, I think, is because they were formerly like a graphics processor company. And the way the silicon kind of arrange itself, align nicely with the algorithms used in AI. So there's a synergy between sort of the mathematics behind AI, the silicon used to implement the algorithms. So those sort of three things, the math, the software algorithms, and the silicon came together to propel Nvidia forward. It's interesting, I was talking one, like. The actual hardware to create the chip. Because something's doing the crunching. Okay. And it's silicon. Right. Well, that's. I was talking to some tech guy in New York, there's, earlier this week about how there's. It's not Moore's law, it's another thing. But there's only so much density we can put on these chips. Yeah, right. You know, and silicon can only be so fast. Like, we basically have to create a new element for, you know, chips to become faster down the road, or it's gonna not be able to. We're. It's like a logarithmic line. It's not exponential. It's like it starts to peak and you get a flat line down. So, like, where technology, speed of light. Is the fastest you can go to communicate data. But some of the graphics processors achieve speed by processing in parallel. So you have multiple sub processors within the chip to split the data up into pipelines, process those pipelines together, and then spit out the output. And that's the genius behind the Nvidia technology. Did you know that the new chips everyone's talking about with Nvidia are like 10,000 pounds? Or like a thousand pounds? I did, like, like 10ft long. They're like, big. And I didn't know this. The data center, like, these chips and data centers, like, huge. Yeah. That's how powerful these are. And we talked about that, about, like, how much energy it's gonna take to power AI in the future. It's probably partially the weight is partially the heat sink. Yeah, probably. Yeah, exactly. The cooling system. No question. That's right. All right, well, Mike, thanks for coming on for this specific podcast talking about AI around it. I know we're next one here in a couple days. We're getting to public sector, private sector jobs around kind of, you know, the patent world and displacement, things like that. Also, talk about some EV's here in a couple podcasts, but we'll get to that next time. Thanks for everyone for tuning in. We'll see you next time. Thank you for having me. The opinions expressed in the podcast are for general informational purposes only and are not intended to provide specific advice or recommendations for any investment, legal, financial, or tax strategy. It is only intended to provide education about the financial industry. Please consult a qualified professional about your individual needs.